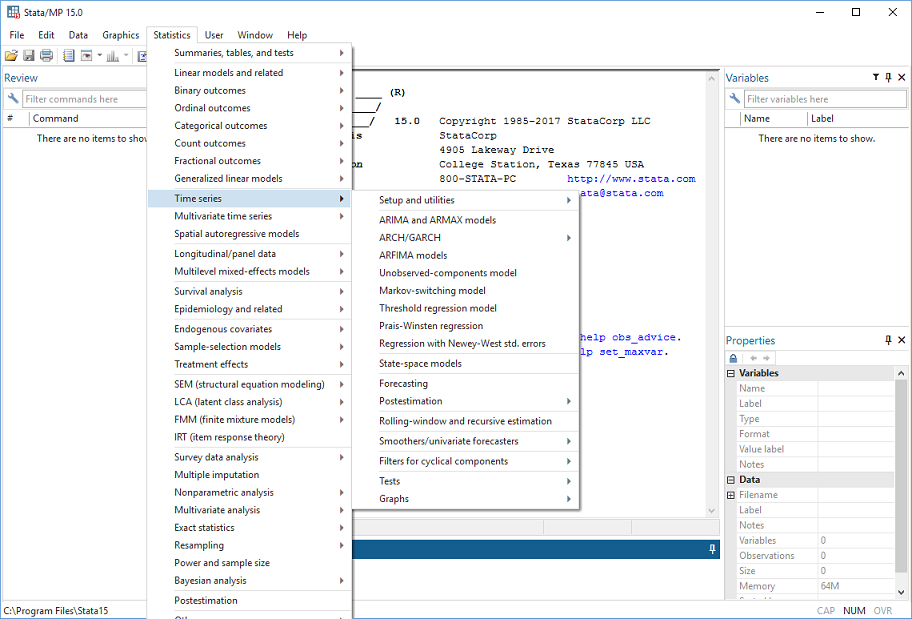
Exponential smoothing is a rule of thumb technique for smoothing time series data using the exponential window blogger.coms in the simple moving average the past observations are weighted equally, exponential functions are used to assign exponentially decreasing weights over time. It is an easily learned and easily applied procedure for making some determination based on prior Help Center; less; Download Free PDF. Download Free PDF. Hill, Griffiths, Lim Principles of Econometrics. Seda Mkrtchyan. Download Download PDF. Full PDF Package Download Full PDF Package. This Paper. A short summary of this paper. 27 Full PDFs related to this paper. Read Paper. Hill, Griffiths, Lim Principles of Econometrics million+ members | Manage your professional identity. Build and engage with your professional network. Access knowledge, insights and opportunities
Exponential smoothing - Wikipedia
Exponential smoothing is a rule of thumb technique for smoothing time series data using the econometrics assignment help stata window function. Whereas in the simple moving average the past observations econometrics assignment help stata weighted equally, exponential functions are used to assign exponentially decreasing weights over time.
It is an easily learned and easily applied procedure for making some determination based on prior assumptions by the user, such as seasonality. Exponential smoothing is often used for analysis of time-series data, econometrics assignment help stata.
Exponential smoothing is one of many window functions commonly applied to smooth data in signal processingacting as low-pass filters to remove high-frequency noise. This method is preceded by Poisson 's use of recursive exponential window functions in convolutions from the 19th century, as well as Kolmogorov and Zurbenko's use of recursive moving averages from their studies of turbulence in the s.
The use of the exponential window function is first attributed to Poisson [2] as an extension of a numerical analysis technique from the 17th century, and later adopted econometrics assignment help stata the signal processing community in the s. Here, exponential smoothing is the application of the exponential, or Poisson, window function. Exponential smoothing was first suggested in the statistical literature econometrics assignment help stata citation to previous work by Robert Goodell Brown in[3] and then expanded by Charles C.
Holt in Simple exponential smoothing is easily applied, and it produces a smoothed statistic as soon econometrics assignment help stata two observations are available. Sometimes the statistician's judgment is used to choose an appropriate factor. Unlike some other smoothing methods, such as the simple moving average, this technique does not require any minimum number of observations to be made before it begins to produce results, econometrics assignment help stata.
To accurately reconstruct the original signal without information loss all stages of the exponential moving average must also be available, because older samples decay in weight exponentially. This is in contrast to a simple moving average, in which some samples can be skipped without as much loss of information due to the constant weighting of samples within the average.
If a known number of samples will be missed, one can adjust a weighted average for this as well, econometrics assignment help stata, by giving equal weight to the new sample and all those to be skipped, econometrics assignment help stata.
This simple form of exponential smoothing is also econometrics assignment help stata as an exponentially weighted moving average EWMA. Technically it can also be classified as an autoregressive integrated moving average ARIMA 0,1,1 model with no constant term. Because exponential smoothing requires that at each stage we have the previous forecast, it is not obvious how to get the method started.
We could assume that the initial forecast is equal to the initial value of demand; however, this approach has a serious drawback. Exponential smoothing puts substantial weight on past observations, so the initial value of demand will have an unreasonably large effect on early forecasts.
This problem can be overcome by allowing the process to evolve for a reasonable number of periods 10 or more and using the average of the demand during those periods as the initial forecast. For every exponential smoothing method we also need to choose the value for the smoothing parameters. For simple exponential smoothing, there is only one smoothing parameter αbut for the methods that follow there is usually more than one smoothing parameter.
There are cases where the smoothing parameters may be chosen in a subjective manner — the forecaster specifies the value of the smoothing parameters based on previous experience. However, a more robust and objective way to obtain values for the unknown parameters included in any exponential smoothing method is to estimate them from the observed data.
The unknown parameters and the initial values for any exponential smoothing method can be estimated by minimizing the sum of squared errors SSE. Hence we find the values of the unknown parameters and the initial values that minimize. Unlike the regression case where we have formulae to directly compute the regression coefficients which minimize the SSE this involves a non-linear minimization problem and we need to use an optimization tool to perform this.
The name 'exponential smoothing' is attributed to the use of the exponential window function during convolution. By direct substitution of the defining equation for simple exponential smoothing back into itself we find that. A geometric progression is the discrete version of an exponential functionso this is where the name for this smoothing method originated according to Statistics lore. Exponential smoothing and moving average have similar defects of introducing a lag relative to the input data.
While this can be corrected by shifting the result by half the window length for a symmetrical kernel, such as a moving average or gaussian, it is unclear how appropriate econometrics assignment help stata would be for exponential smoothing.
They differ in that econometrics assignment help stata smoothing takes into account all past data, whereas moving average only takes into account k past data points. In the signal processing literature, econometrics assignment help stata, the use of non-causal symmetric filters is commonplace, and the exponential window function is broadly used in this fashion, but a different terminology is used: exponential smoothing is equivalent to a first-order infinite-impulse response IIR filter and moving average is equivalent to a finite impulse response filter with equal weighting factors.
Simple exponential smoothing does not do well when there is a trend in the data. Econometrics assignment help stata nomenclature is similar to quadruple exponential smoothing, which also references its recursion depth. This slope component is itself updated via exponential smoothing. One method, works as follows: [13]. Double exponential smoothing is given by the formulas. A second method, referred to as either Brown's linear exponential smoothing LES or Brown's double exponential smoothing works as follows, econometrics assignment help stata.
where a teconometrics assignment help stata, the estimated level at time t and b tthe estimated trend at time t are:. Triple exponential smoothing applies exponential smoothing three econometrics assignment help stata, which is commonly used when there are three high frequency signals to be removed from a time series under study.
There are different types of seasonality: 'multiplicative' and 'additive' in nature, much like addition and multiplication are basic operations in mathematics. If every month of December we sell 10, more apartments than we do in November the seasonality is additive in nature.
Multiplicative seasonality can be represented as a constant factor, not an absolute amount. Triple exponential smoothing was first suggested by Holt's student, Peter Winters, in after reading a signal processing book from the s on exponential smoothing. The use of a triple application is considered a rule of thumb technique, rather than one based on theoretical foundations and has often been over-emphasized by practitioners.
Triple exponential smoothing with multiplicative seasonality is given by the formulas [1]. From Wikipedia, the free encyclopedia. Generates a forecast of future values of a time series. It has been suggested that Exponential moving average be merged into this article. Discuss Proposed since June Retrieved 23 May Digital Signal Processing.
Prentice Hall, econometrics assignment help stata. ISBN Exponential Smoothing for Predicting Demand. Cambridge, Massachusetts: Arthur D. Little Inc. Office of Naval Research Memorandum, econometrics assignment help stata. reprinted in Holt, Charles C. January—March International Journal of Forecasting. doi : Smoothing Forecasting and Prediction of Discrete Time Series.
Englewood Cliffs, NJ: Prentice-Hall. Single Exponential Smoothing". Retrieved 5 July Retrieved 26 July Page Production and Operations Analysis 6th ed. SAP AG. Retrieved 23 January Double Exponential Smoothing". Retrieved 25 September Retrieved 23 June April Management Science. Retrieved 5 June Archived from the original on 16 July Outline Index. Descriptive statistics.
Variance Standard deviation Average absolute deviation Coefficient of variation Percentile Range Interquartile range. Central limit theorem Moments Skewness Kurtosis L-moments. Index of dispersion. Grouped data Frequency distribution Contingency table.
Pearson product-moment correlation Rank correlation Spearman's ρ Kendall's τ Partial correlation Scatter plot. Bar chart Biplot Econometrics assignment help stata plot Control chart Correlogram Fan chart Forest plot Histogram Pie chart Q—Q plot Run chart Scatter plot Stem-and-leaf display Radar chart Violin plot. Data collection. Population Statistic Effect size Statistical power Optimal design Sample size determination Replication Missing data.
Sampling stratified cluster Standard error Opinion poll Questionnaire. Scientific control Randomized experiment Randomized controlled trial Random assignment Blocking Interaction Factorial experiment. Adaptive clinical trial Up-and-Down Designs Stochastic approximation. Cross-sectional study Cohort study Natural experiment Quasi-experiment. Statistical inference. Population Statistic Probability distribution Sampling distribution Order statistic Empirical distribution Density estimation Statistical model Model specification L p space Parameter location scale shape Parametric family Likelihood monotone Location—scale family Exponential family Completeness Sufficiency Statistical functional Bootstrap U V Optimal decision loss function Efficiency Statistical distance divergence Asymptotics Robustness, econometrics assignment help stata.
Estimating equations Maximum likelihood Method of moments M-estimator Minimum distance Unbiased estimators Mean-unbiased minimum-variance Rao—Blackwellization Lehmann—Scheffé theorem Median unbiased Plug-in.
Quantile Regression in Stata
, time: 11:52Sign Up | LinkedIn

Exponential smoothing is a rule of thumb technique for smoothing time series data using the exponential window blogger.coms in the simple moving average the past observations are weighted equally, exponential functions are used to assign exponentially decreasing weights over time. It is an easily learned and easily applied procedure for making some determination based on prior Help Center; less; Download Free PDF. Download Free PDF. Hill, Griffiths, Lim Principles of Econometrics. Seda Mkrtchyan. Download Download PDF. Full PDF Package Download Full PDF Package. This Paper. A short summary of this paper. 27 Full PDFs related to this paper. Read Paper. Hill, Griffiths, Lim Principles of Econometrics million+ members | Manage your professional identity. Build and engage with your professional network. Access knowledge, insights and opportunities
No comments:
Post a Comment